Meta-analysis
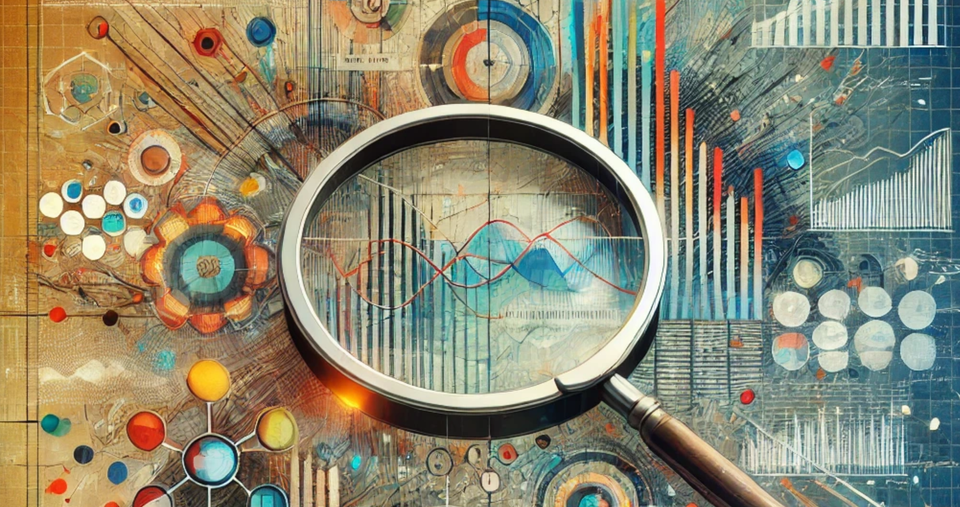
The name is synonymous with 'solid evidence' but what exactly is it?
Meta-analysis is process of combining the results of different (randomised) trials to derive a pooled estimate of effect.
Meta-analysis is a statistical method that looks at a whole bunch of other studies that have in turn all looked at the same thing.
The idea is that by joining lots of smaller studies together, you get around the fact that they're not powerful enough to deduce any meaningful conclusions on their own.
How is it different to a systematic review?
- "A systematic review answers a defined research question by collecting and summarising all empirical evidence that fits pre-specified eligibility criteria."
- "A meta-analysis is the use of statistical methods to summarise the results of these studies."
The process
- Decide what question or problem you're going to attack
- Come up with a hypothesis
- Do an exhaustive literature review to find everyone else who's attempted to answer the same question
- Decide whether you can use these studies by comparing them to your predefined inclusion criteria (sample size/blinding/randomisation)
- Preferably get multiple people to agree on which studies are to be included
- Do some stats and figure out what the effect size of each study is - bigger study = bigger effect size
- Summarise and present the results using pretty graphs and something called a forest plot.
The benefits
- It's a reliably objective and quantitative appraisal of the available evidence
- Increased power - small individual studies often lack sufficient power to draw any meaningful conclusions, so combining multiple studies can get around this issue - this means a meta-analysis has a lower risk of a false negative result
- Increased precision - you getter a better idea of actual effect sizes as a result of a much bigger combined sample size
- Summary of the literature - the process of meta-analysis necessitates reviewing as much of the literature as possible, which brings multiple separate avenues of research together and can help identify other areas for exploration
- Patterns - even if you can't conclude anything meaningful, meta-analysis can often identify trends or patterns that might stimulate interest in further research
- Can help guide future research
The downsides
- The studies are often all different in their inclusion criteria, what they're actually measuring as an outcome and their methodology, which can make comparing them rather hard work
- You might not include all the relevant studies, either through not searching correctly, inappropriately discounting them or not seeing them at all because they were published in obscure journals
- Publication bias means that studies with an interesting outcome are more likely to get published, so when you're doing a meta-analysis you often don't see the other studies where nothing interesting was found
- Bad studies = bad meta-analysis
- Clinical relevance to the individual patient is not guaranteed, even if you have strong statistical results
- You will have to make some assumptions along the way during your meta-analytic journey, and these might introduce error to the results
- A positive meta-analysis isn't enough to drive a change in practice, and usually still needs confirmation with a large scale randomised controlled trial
Forest Plot
Looks a bit like this:
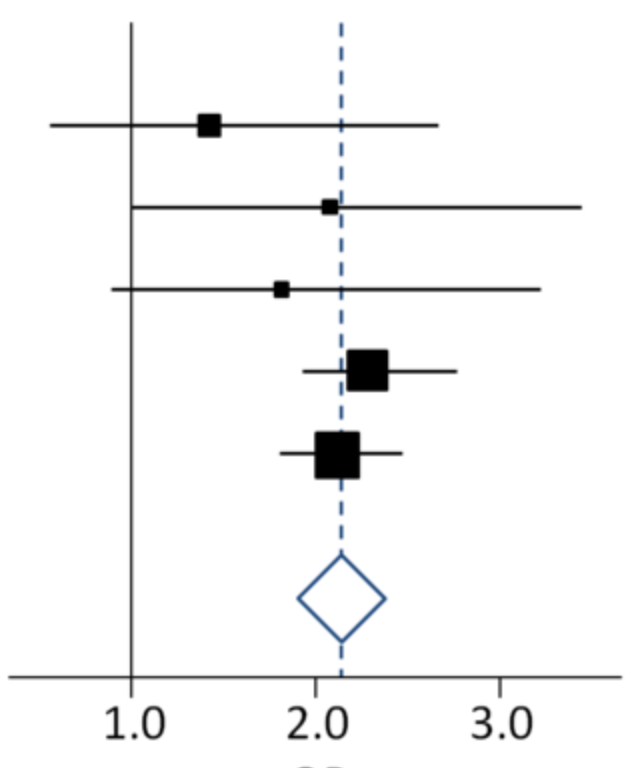
- The diamond is the overall result of the meta-analysis
- Each individual study is included as a box with the sideways arms
- The width of the box represents the standard error of the study or the sample size depending on how you're choosing to draw the plot
- The side arms represent the confidence intervals at 95% (smaller arms = smaller confidence interval)
- If the side arm crosses the vertical line through 1.0 then the study has a non-significant result
- The width of the diamond is the confidence interval of the meta-analysis
- If the diamond doesn't touch the vertical line through 1.0, then the meta-analysis has a significant result
What factors have an effect on the overall result?
- Bigger sample size
- Bigger effect size (box further from vertical line)
- Greater precision (narrower box)
- Smaller confidence interval
An example
Mortality and morbidity after total intravenous anaesthesia versus inhalational anaesthesia: a systematic review and meta-analysis
— eClinicalMedicine – The Lancet Discovery Science (@eClinicalMed) May 21, 2024
Postoperative mortality and organ-related morbidity was similar for intravenous and inhalational anaesthesiahttps://t.co/GlQH2JRQyK pic.twitter.com/1A0pP9Z4n2